Human Capital Data and Financial Outcomes
by Benjamin Zweig
1. Introduction: Human Capital Data
A company is made up of many things, all coming together to create value that can ultimately be captured by investors. Understanding the components of a company is a critically important part of determining its value, a core activity of financial analysts and investors. For many years, financial researchers have built increasingly sophisticated models of valuation. The recent trend toward analyzing non-traditional or “alternative” data related to companies has only served to improve the understanding of company performance. While all of this represents positive advancements in financial sophistication, the largest and most important part of every business has been almost entirely ignored. This piece, of course, is human capital . Human capital is the talent within an organization, the employees of a company that do everything from building technology to allocating resources to selling products and creating revenue. The aggregate share of costs attributable to human capital is two thirds. That is an astounding number. For an average company, people comprise two-thirds of its expenses and two thirds of the value derived from the company are attributable to people. This share has been remarkably stable for many decades. For many modern companies, this share is far higher and only promises to increase as the economy continues to grow more intangible.
Because of the pervasive nature of employees within a company, understanding the employee dynamics can really lead investors to better understand all the dynamics of a company. There is no part of a company, a strategy or a technology, that cannot be well represented through employees. The ability to judge an organization on talent seems to be well understood by pockets of the economy outside of Wall St. Even in the public sector discourse, “Personnel is policy” is a common trope used to imply that the management of an organization is only as good as the people who run it – this is as true for companies as it is for governments.
If human capital comprises the lion’s share of company value, it is truly surprising that it has never been truly rigorously analyzed by mainstream investors. The main reason why this has persisted for so long is that data on employees has been inaccessible for so long. There was never an accounting mandate for companies to release data on human capital in the same way that they were required for physical capital. Thanks to the possibility of gathering large scale web data, this no longer matters. Alternative data vendors such as Thinknum have gathered large amounts of human capital data which allows investors to analyze the workforce dynamics of thousands of companies. Because of this capability, investors will no longer be constrained to getting a full picture of what is happening within a company.
2. Data: Job Postings
The earliest leading indicator of human capital trends is surely job postings. The patterns of job postings can be predictive of budgets, revenues, and market value. As job posting data get analyzed at finer and infer levels, patterns of a company’s workforce become revealed which can indicate changes to a company’s strategy.
The purpose of this study is to use job posting data, provided by Thinknum, to explore relationships between job postings and company performance. This study is preliminary by design. There are many ways to analyze this data and I hope this report can serve as a jumping off point for others to do more advanced analyses.
Company performance data comes the Capital IQ databases of Compustat, from S&P. They are merged with the Thinknum data using either Stock Ticker or CUSIP.
The final dataset tracks 1957 public companies for each quarter in 2016, 2017, and 2018.
The four variables included are job posting volume, expense, revenue, and market cap. Derivations of these variables are used over the course of the analysis.
3. Trends & Relationships
The most basic exploration is to look for simple relationships between job posting volume and financial outcomes. Even this, however, needs some careful tweaking. Because the data is panel/longitudinal in nature, and tracks many companies over multiple time periods, it is necessary to isolate the cross-sectional relationships between the variables of interest with the time-series relationships. The cross-sectional relationships are largely uninformative – of course companies with more job postings are bigger companies with high values of expense, revenue, and market value.
In order to capture only the time-series relationships, a fixed effects model must be used. That way, we can see how job postings volumes within a company over time track with fundamental outcomes within a company over time . In order to not give outsize weight to large companies with high variance in job posting volume, it is important to consider percent changes rather than nominal changes. For that reason, elasticities were measure between job postings and three fundamental variables.
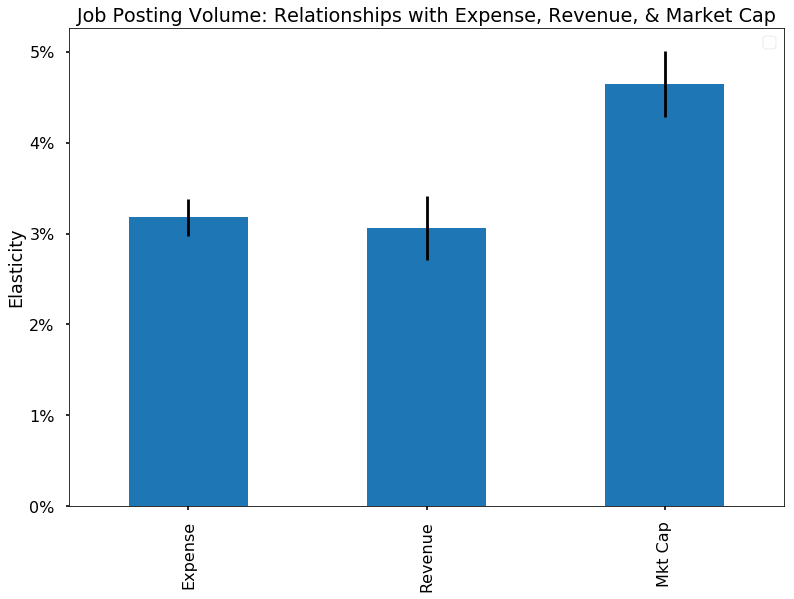
As shown in chart 1, all elasticities are positive and strongly statistically significant.
The most intuitive relationship is that with expense. Job postings represent the plan to hire more employees which, if hired, will contribute to expense. The relationship with revenue appears roughly the same as the relationship with expense which shows that the employees being hired contribute to the business in a way that tracks closely with cost (indeed, if the magnitude were very different, an arbitrage opportunity may arise). The relationship with market cap is strongest of all because hiring employees represents growth. It could be that hiring sends a signal of growth or that growth enables and necessitates hiring – either way, the predictive power is very strong.
4. A Leading Indicator
Although it is clear that job posting volume does correlate with fundamental variables, the question remains as to what predictive power a change in the volumes would imply for the fundamentals of a company. The relationship between employees, expense, and revenue is complicated by the reality that employees contribute to expense immediately but may not produce value for a long time.
For this analysis, we take a look at the relationship between a change in job posting volume with a change in expense and revenue in subsequent quarters.
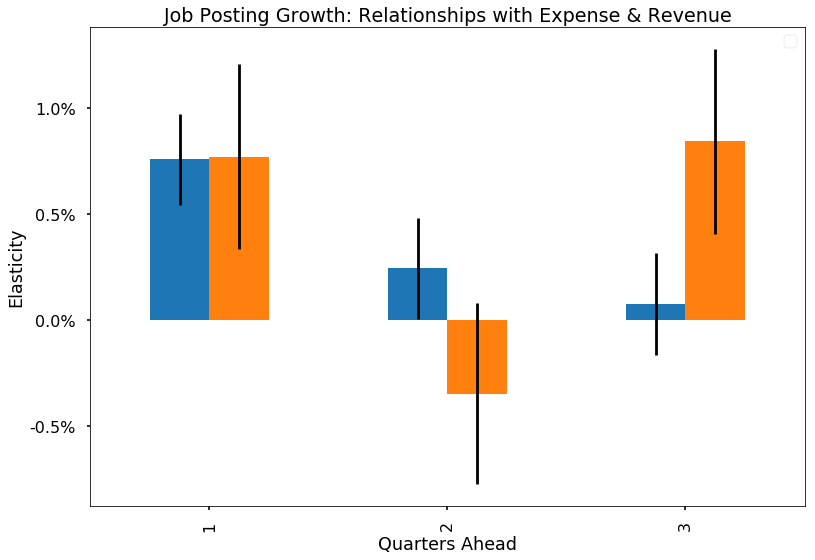
In Chart 2, we see the dynamics of the impacts of job posting growth. If there is a jump in job posting volume, it will likely take some for the jobs that have been posted to get filled. That will result in growth in expense in the following quarter. The impact on expense quickly erodes over time, however, because there is a constant flow of attrition that eats away at the employee base. The impact on revenue is more complex. The impact is strongest in one quarter from the postings, statistically indistinguishable from zero two quarters out and strong again three quarter out. There could be multiple reasons for this but the way that I make sense of it is that the impact in the first quarter is due to revenue that was foreseen at the time of the posting – it was this expected revenue that companies had planned to pay for the new employees. The third quarter is likely when the employees hired actually have a positive causal impact on revenue.
5. Extensions
Thus far, we’ve only explored aggregate job postings as the variable we’re concerned with. However, jobs are multidimensional, with different roles, seniority levels, skills, backgrounds, locations, etc. In this section, I slice the data by seniority levels and roles to see if the relationships expense, revenue, and market differ for different types of postings.
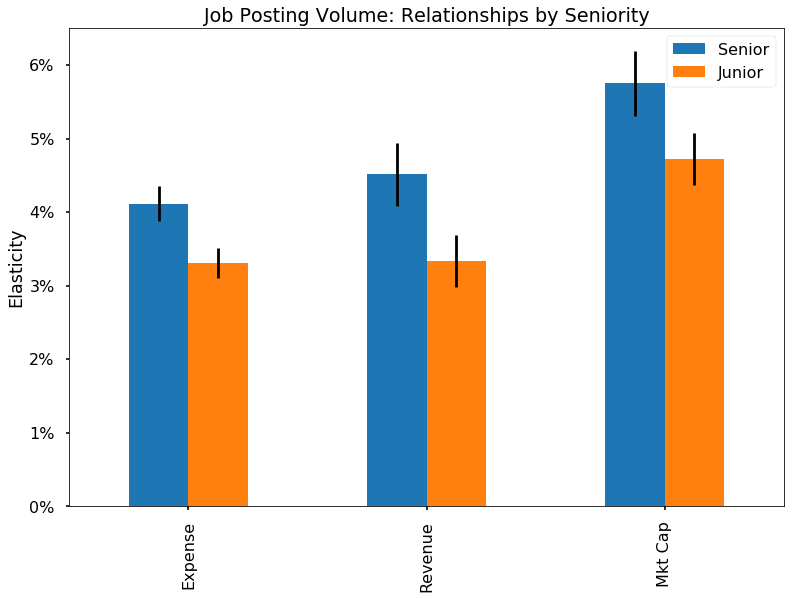
In Chart 3, we see that relationships are stronger across the board for more senior hires. To segment data this way, I filtered by titles with senior-related words in their titles and those without . 1
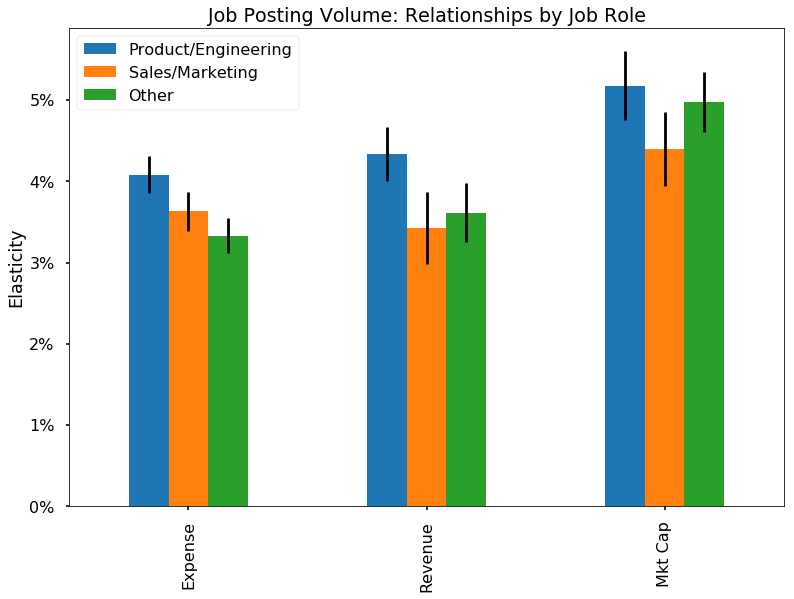
In Chart 4, it appears that product and engineering roles have the greatest association with financial outcomes while sales and marketing roles do not seem to have as positive of an impact. To segment data this way, I filtered by titles with product/engineering words, titles with sales/marketing words, and those without.
6. Discussion
These analyses, more than anything, are meant to whet the appetite and entice others to find interesting relationships between human capital metrics and financial outcomes. It is not plausible that such an enormous part of what comprises a company could not yield large insights about where a company is headed and how it will likely perform. Indeed, some interesting relationships were also found by others between attrition rates and financial performance.
What Thinknum and others provide is a foundation for understanding companies in more complete ways than ever before. The largest and most important area that needs to be analyzed rigorously is that of human capital. Because of the simple magnitude of the expense and revenue driven by talent, it is likely the area of valuation that is hiding the most uncovered alpha. Those who do not rigorously analyze talent dynamics simply cannot rigorously analyze a company.
There is a long way to go to develop rigorous and scientific ways to analyze human capital. The almost endless potential represents an opportunity not just for financial researchers but for the broader economy. If equity markets respond to workforce dynamics, that puts pressure on company executives to develop clear and coherent talent strategies that will ultimately lead to an improved allocation of labor in the economy, improved information about the supply and demand for skills and occupations, more accountability of managers, and a more engaged workforce.
About the Author:
Ben Zweig is an Adjunct Professor at NYU Stern School of Business where he teaches data analytics. He holds a PhD in Economics and works at the intersection of labor economics and alternative data.
Acknowledgements:
I’d like to thank my research assistant, Yu-Ping Lin who was invaluable in analyzing this data.
Gabe Gleason, from the Thinknum team, was incredibly generous in helping out with data demos, access, and everything else required to get up and running quickly.